Analisis Sentimen terhadap Kebijakan Food Estate Menggunakan Algoritma Support Vector Machine
Abstract
The food estate policy has become a key topic in public discussions in Indonesia regarding food security. However, its implementation has sparked reactions on social media, ranging from positive to negative and neutral. This study aims to analyze public sentiment towards the food estate policy using the Support Vector Machine (SVM) algorithm. SVM was chosen for its proven effectiveness in text classification, and previous studies have demonstrated high accuracy in sentiment analysis. Data were collected from the social media platform X using scraping techniques, followed by data processing. The processed data were then classified into three sentiment categories (positive, negative, and neutral) using SVM with linear, RBF, polynomial, and sigmoid kernels. The eval__uation results show that SVM with a linear kernel and parameter C=2 provided the best performance, achieving 79% accuracy, 80% precision, 79% recall, and an F1-score of 79%. These findings indicate that SVM is capable of accurately classifying public sentiment, offering valuable insights for policymakers in eval__uating the social impact of the policy.
Downloads
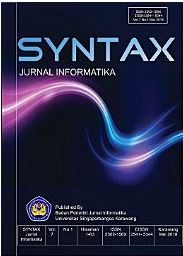